An Introduction To Eigenvectors & Eigenvalues Towards Quantum Computing
Learn the mathematical basis of quantum measurements by performing them by hand.
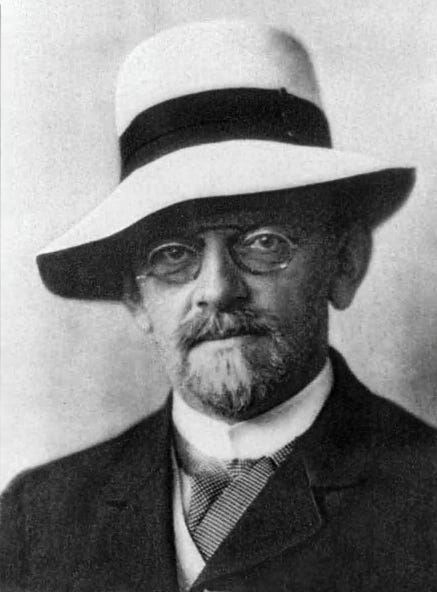
Eigenvectors & Eigenvalues are two important terms you will encounter multiple times when reading about Quantum Computing.
These terms are crucial to understand, and this lesson is all about them.
Let’s do this step by step.
Keep reading with a 7-day free trial
Subscribe to Into Quantum to keep reading this post and get 7 days of free access to the full post archives.